TADEEP: Traducción automática en profundidad
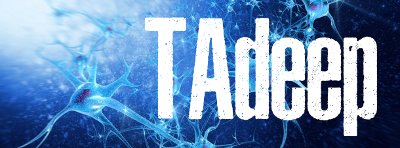
Kalitatezko Itzulpen Automatikoa (IA) jarraitzen zuen erronka izaten 2015ean. Enpresa erabiltzaileek eta erabiltzaile partikularrek ezagun zituzten IAren abantailak eta bazekiten zeintzuk ziren erabileraren mugak. Enpresek produktibitatea handitu nahi zuten, IAko erremintak eta postedizio-inguruneak konbinatuz. Partikularrek intentsiboki zerabiltzen IAa nahiz eta hark eskaintzen zuen kalitatea beti ez izan lortu nahi zutena. IXA taldeak TACARDI eta QTLeap proiektuetako emaitzetan oinarrituta artearen egoera hobetuko zuten tekniketan ikertzea proposatzen zuen bi alderditan:
(1) Analisi sakonean eta ikasketa sakonean oinarritutako IA. "word embedding" eta "deep-learning" bidez.
(2). Domeinu espezifikoetan egokitutako IA. Domeinu baterako egindako egokitze on bat kalitate-hobekuntzarako berme onenetako bat da: QTLeap proiektuko informatikako domeinu teknikoetan komertzialki interesgarriak diren hobekuntzak lor zitezkeen, baita TACARDI proiektuko sare sozialetan ere, edo gaurkotasun handiko domeinu medikoan edo kontsumokoan.
Bukateran, proiektuaren emaitzak aplikatu ziren https://www.modela.eus itzultzaile automatikoan hasieran, eta hori gero https://itzultzailea.eus eta https://lingua.eus/ itzultzaileetan ere izan. Proiektuaren emaitzak EGOKI moduan ebaluatu zuen Ministerioak 2020ko ekainean. TADEEP proiektu hau bukatzean taldeak DOMINO proiektua (http://ixa2.si.ehu.es/domino) lortu zuen Ministerioan, Santiagoko Unibertsitateko Pablo Gamallo ikerlariaren lankidetzarekin.
In 2015, high quality machine translation (MT) was still a challenge. Users, whether companies or individuals, were currently aware of the benefits and limitations of these systems. Whereas companies focused on increasing productivity by combining translation memories, CAT tools and post-editing environments, regular users used MT systems extensively even when the quality did not reach the desired level.
Based on our previous work and results in the TACARDI project (MINECO-lTIN2012-38523-C02-01) and the QTLeap European project (FP7-ICT-2013.4.1-610516), we proposed to investigate techniques that improve the state of the art of MT systems by focusing on two important aspects:
(1) Deep analysis and Deep NLP. Neural networks and their application through "word embedding" and "deep-learning" had revolutionized the area of NLP in the last three years.
(2) Domain-specific MT. Given their current level of output quality, appropriate domain adaptation was the best guarantee for quality improvement: technical domains, such as the IT domain explored in the QTLeap project, social networks explored in the TACARDI project, or other highly topical domains such as the medical domain or services can achieve improvements of commercial value.
The working languages of the project were mainly English, Spanish and Basque. The first two avail of large quantities of information to exploit during research and they have high possibilities to reach the market. Basque, in turn, poses a research challenge given its rich morphology, free word order and fewer available resources, which presents an ideal set-up to explore the generalisability of the project's outcomes to other language pairs.
The IXA group at UPV/EHU had the know-how and experience required to undertake this project. The group did not only include experts in MT but also experts in morphology, syntax, semantics and machine learning.
The results of this projects were initially applied to the automatic translator MODELA (https://www.modela.eus) and later to the https://itzultzailea.eus and https://lingua.eus/ translators. The project results were evaluated as SATISFACTORY by the Ministry in June 2020. At the end of this TADEEP project, the team obtained in the Ministry the DOMINO project (http://ixa2.si.ehu.es/domino), in collaboration with Pablo Gamallo, researcher of the University of Santiago .